Vineel Rayapati
Data Scientist
Preserving the Trails for Tomorrow
America’s magnificent national parks have long captured the imagination of outdoor enthusiasts eager to experience nature's majesty. Yet this enthusiasm has brought huge crowds that strain park resources. As the National Park Service (NPS) marks its 100th anniversary in 2016, addressing issues around sustainability and capacity management remains paramount for the longevity of these cherished public lands. Forecasting future hiking trail visitation provides data-driven insights to help NPS officials strategically allocate staffing, amenities, permits and promotional efforts to align with projected usage across hundreds of trails each season.
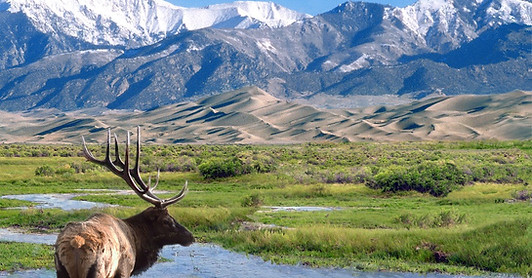
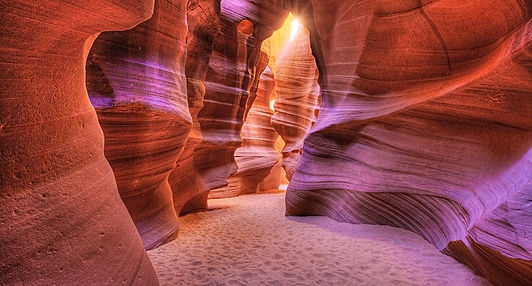
Historic attendance figures reveal staggering growth at many top parks like Grand Canyon, Yellowstone and Yosemite, fueled by factors like social media promotion, expanded international tourism, and the health benefits of outdoor recreation. Balancing conservation, access and traffic flow requires extensive coordination. By leveraging visit data analytics, park managers can get ahead of trends at individual trails instead of reacting after congestion or infrastructure issues emerge. Advanced forecasting techniques utilizing machine learning provide more accurate models compared to traditional linear regression methods.
Specifically, time series forecasting examines sequential data over time to uncover seasonal, cyclical and noise patterns. Sophisticated autoregressive models called ARIMA (Auto-Regressive Integrated Moving Average) are well suited to handling time-based information like visit metrics and have become a leading method used by data scientists. By tuning parameters and feeding these algorithms historical figures, reliable estimates of future trail usage can be generated even years out. The predictive capabilities vastly improve planning agility for NPS officials facing complex decisions with limited resources across expansive natural areas most have never physically visited.
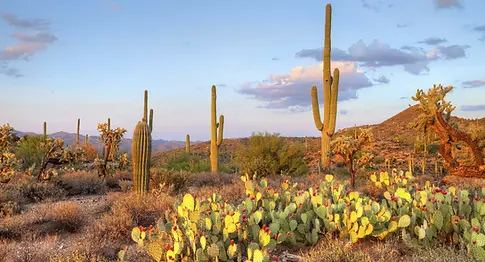

Such data science applications align with the NPS goal to “enhance the NPS’ capability to manage parks and programs through strategic use of information”. While visit data offers but one component to consider, it directly showcases public demand signaling where proactive measures may help. Combining trail traffic forecasts with factors like acreage, facilities, and ecology facilitates recommendations on permitting limits, facilities upgrades, events scheduling, bio-diversity protection and more. Of course, any technology solution requires buy-in from park stakeholders so transparency and interpretability are key.
In sum, creating accurate trail visitation forecasts empowers park officials to progress their conservation mission amidst record outdoor recreation demand. Just as weather predictions allow society to take proactive measures for storms, visitation analytics prevents scenarios where human enthusiasm overwhelms environmental priorities. The famous naturalist John Muir who helped establish the National Park Service recognized that “Everybody needs beauty...places to play in”. Data science helps ensure these places endure by aligning community connections with sustainable capacity. This project showcases how machine learning can strengthen society’s relationship with nature instead of detracting humanity from its gifts.
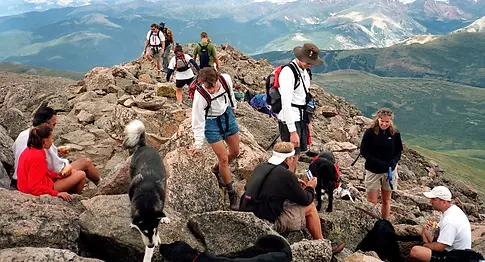
Motivation for the national park visitation forecasting
Accurate forecasts of future visitation levels allow national parks to efficiently plan staffing, infrastructure, and resource allocation to meet demand. Modeling expected attendance would provide parks financial estimates for budgeting around revenues from entry fees, concessions, and permits. Understanding potential visitation impacts would let parks assess environmental, ecological, and usage impacts on facilities and lands to shape sustainability initiatives and upgrades. Local communities could use the forecasts to better estimate the tourism economic impacts that major drivers like park attendance bring to hotels, restaurants, tour companies, and partners. Marketing groups can even develop promotions and messaging to target lower-visitation periods. In summary, data-driven visitation forecasts would facilitate resource planning, revenue management, impact control strategies, tourism economics, marketing campaigns, and operational readiness for emergencies at national parks. Quantitative modeling to predict park attendance outlines stakeholder opportunities around budgeting, capacity planning, sustainability, promotion, and ensuring excellent visitor experiences are maintained. Accurate future estimates allow national parks and partners to make better informed decisions through forecasting models.
Previous relevant works on
forecasting national park visitation
Prior studies have demonstrated using time series and machine learning techniques to model tourism demand for national parks. A 2012 thesis employed ARIMA models to forecast monthly Muir Woods visitation up to a year ahead, finding seasonal ARIMA configurations outperformed baseline predictions. Academics in 2018 tested machine learning approaches like LSTM and random forests to predict monthly Yellowstone attendance, determining neural networks provided greater accuracy. Building on this, a 2019 paper combined Yellowstone’s lagged visitation data with climate variables through a stacked LSTM architecture to capture seasonal effects. More recently, research has turned to aggregated models like multi-branched LSTMs predicting foreign arrivals countrywide rather than for specific parks. On a granular level, Facebook’s open-source Prophet procedure was applied in 2021 to predict international tourism in Barbados across yearly, monthly and weekly seasonality. The collection of studies exemplifies adaptable frameworks using ARIMA, SARIMA, Prophet, LSTM networks, and regression trees to reliably forecast park-level and national tourism volume over monthly to yearly horizons. The data characteristics and prediction goals align closely with the objectives of this project on modeling future national park visitation.
10 questions I would want to explore further with this national park visitation data
-
Which specific parks have seen the largest gains or declines in visitors over the past 5 years? What factors may be driving those shifts?
-
Is there a relationship between increased park popularity and ratings on travel review sites like Yelp or TripAdvisor?
-
How strong is the correlation between search volume for a park and its visitation totals for the same year? Could search data improve visit predictions?
-
Do parks with free entry tend to attract more first-time visitors compared to paid-entry parks based on visitor surveys?
-
What visitation patterns emerge when analyzing visitor home locations and primary travel routes to different parks?
-
How do visit rates for camping, lodging, day-use, etc. compare across park regions and over time?
-
Are international visitor numbers growing or shrinking across major parks that report international attendance?
-
How extreme is the variation in visitor counts for high-peak versus low-trough dates within each park seasonally?
-
Which visitor segments based on age, group type and activity preference have become more or less represented over the last decade?
-
What trends emerge from sentiment analysis of visitor reviews and experience ratings over recent years for the most visited parks?