Vineel Rayapati
Data Scientist
The Buzz Behind the Numbers
The stock market is a dynamic and ever-changing landscape where millions of transactions are made daily. Behind these transactions lies an intricate web of human decision-making, influenced by everything from corporate earnings reports to global events. In this interconnected world, news plays a pivotal role in shaping investor sentiment. Headlines about economic policies, technological breakthroughs, or geopolitical tensions can significantly sway stock prices. This project focuses on uncovering the relationship between financial news sentiment and stock price movements, offering a fresh perspective on how public perception shapes market behavior.


Stock prices are not driven solely by financial metrics such as revenue, profit, or earnings per share. The mood and sentiment surrounding these numbers, as reflected in news articles, play an equally vital role. For example, a positive article about a company’s innovative product launch can trigger enthusiasm among investors, leading to a surge in stock prices. Conversely, negative press about legal troubles or market downturns can instill fear and cause sharp declines. This interplay between sentiment and stock price underscores the need for tools that can interpret the tone of financial news and translate it into actionable insights.
The significance of understanding this relationship extends beyond individual investors. Large financial institutions, hedge funds, and even regulators can benefit from insights into how sentiment impacts the market. Predicting stock prices based on news sentiment has applications in portfolio management, risk assessment, and trading strategies. It empowers market participants to navigate uncertainty with greater confidence, ensuring more informed decision-making. This project explores the potential of machine learning and sentiment analysis in providing these insights, marking a step forward in the intersection of finance and artificial intelligence.
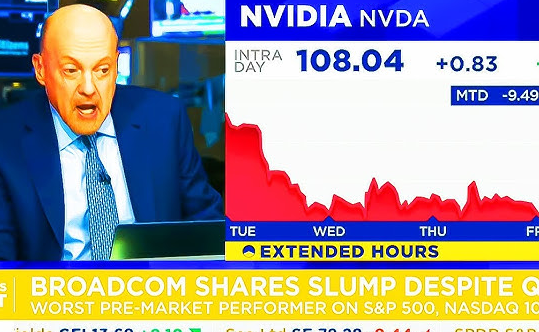
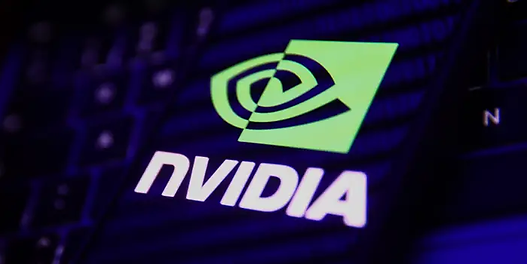
In this project, we combine financial news data with historical stock performance to build predictive models using neural networks. By leveraging tools such as natural language processing and deep learning, this study examines how sentiment scores derived from news headlines correlate with stock price fluctuations. Specifically, the project focuses on Nvidia, a leading technology company, to showcase the power of sentiment-driven predictions. The goal is to bridge the gap between qualitative data (news headlines) and quantitative outcomes (stock prices), offering a unique way to interpret market trends.
The implications of this study are vast. For retail investors, it offers an accessible way to better understand market movements. For financial institutions, it provides a competitive edge in predicting trends ahead of the market. And for regulators, it sheds light on how media influences trading behavior, potentially informing policies to ensure market stability. By exploring the emotional side of economics, this project demonstrates how technology can make sense of complex human behaviors in financial markets. It is a testament to how data science can transform how we view and interact with the financial world.
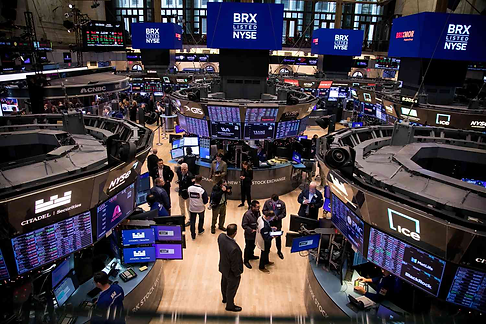
Why Sentiment Matters: The Driving Force Behind Stock Market Movements
Predicting stock prices using news sentiment offers transformative insights for investors, institutions, and regulators in the ever-evolving financial landscape. For individual investors, it provides a clearer understanding of market trends, empowering smarter investment decisions. Financial institutions and hedge funds gain a competitive edge by integrating sentiment analysis into trading algorithms, allowing swift responses to market-moving news. Companies like Nvidia can monitor public perception and tailor strategies, while regulators can analyze how media impacts market behavior to craft policies that ensure transparency and stability. Sentiment-driven predictions also mitigate risks, reducing market volatility and fostering trust among participants. By combining neural networks with sentiment analysis, this project bridges the gap between human emotion and quantitative data, creating a powerful tool for informed decision-making. The interplay between information, perception, and stock movements highlights the importance of sentiment analysis, offering actionable insights that drive smarter strategies across the financial ecosystem.
Previous relevant works on
forecasting national park visitation
Previous studies have explored the use of sentiment analysis and machine learning to predict stock market behavior. In 2015, researchers demonstrated the effectiveness of sentiment scores from financial news in forecasting stock price movements. By 2018, LSTM networks were applied to combine historical stock data and sentiment, improving prediction accuracy. A 2020 study advanced this approach using a hybrid CNN-LSTM model, integrating financial indicators with sentiment analysis for daily price forecasting. More recently, FinBERT was employed to quantify the influence of news sentiment on stock volatility. These studies underscore the value of neural networks in sentiment-driven stock prediction.